Generative artificial intelligence is currently a hot topic in the tech startup world, for good reason. Within the past few months there has been an improvement in the quality of outputs from buzzy projects like ChatGPT, DALL-E 2 and Stable Diffusion. These players are all advancing the underlying technologies a clear notch from cool to useful.
The latter two, along with Midjourney, are examples of latent diffusion models, which generate images from text inputs.
Search less. Close more.
Grow your revenue with all-in-one prospecting solutions powered by the leader in private-company data.
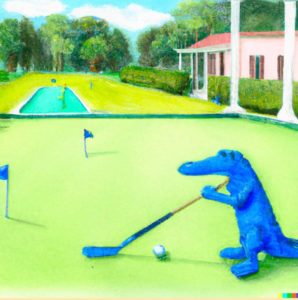
All three models operate in a similar manner in that the user writes a prompt in plain text such as, “watercolor of a blue alligator playing croquet at a country club,” and the model outputs a novel and unique image depicting what the user described.
Consumers got a taste of the power of these models when Lensa went viral in December for turning user uploaded images of themselves into packs of “Magic Avatars,” sets of stylized AI-generated images of the user. When I bought my first pack in December, I received 10 sets of 20 AI-generated images of me, with each set having a particular theme such as“mystical,” “astronaut,” “rock star” and “superhero.”
On the backend, Lensa is deciding which prompts will create interesting image outputs and then standardizing those for each image set. That means the user only needs to upload 10-20 images of themselves to receive the striking, photorealistic images that I am still seeing all over social accounts. While the novelty around these first consumer use cases AI-generated images may already be fading, business applications of latent diffusion models are still in their infancy.
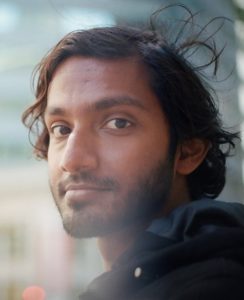
As such, a whirlwind of projects making use of these technologies have popped up in the last weeks and months. As both an early-stage investor at Tuesday Capital and the founder of Moongrove, a company implementing latent diffusion models, I have been giving a lot of thought to which ones make sense. Here are some criteria to consider in weighing whether latent diffusion models make sense for a particular business.
Does a latent diffusion model make sense for my business?
The first is the scale of outputs.
Latent diffusion models are good at generating a large quantity of images, so are inherently a good fit for applications where customers or users want a large quantity of images.
In general, the quality of these images can be good or great, but not perfect. So, almost certainly, the processing pipeline must either have an endpoint that doesn’t require absolute perfection or layers of human checking, editing and polish prior to the final output. Today’s models typically output a fair amount of noise, so if the capacity to review a large number of images is lacking, that use case may not be a good fit.
The second is the need for a high degree of creativity in those outputs. Oftentimes the image generation needs of a business entail iterations of existing content within what can be relatively narrow constraints, such as brand guidelines. Such changes often require small tweaks and subtle changes. While subtlety is not the strength of today’s image models, novelty is. Trying to use the current tools to iterate on existing assets would be akin to hitting a nail with a jackhammer. However, if one is looking for completely novel looks or image backgrounds, today’s technology is ripe. Good examples in the e-commerce space include Zeg and Flair, which can create novel product treatments from words.
Lastly, a good candidate for implementing image-generation models must have highly repeatable workflows and similar types of outputs. Examples of types of outputs include: human faces, product treatments, product packaging, website backgrounds and animated characters. Right now, image outputs are only as good as the text prompts fed to the models, and writing those prompts is still as much art as science. Therefore, to generate a reasonably high quality of outputs, it is best if a business use case involves generating many of the same type of output.
I should note that these criteria are very much for today, right now. I expect, and frankly hope, these points to be dated very soon. This space is rapidly evolving and many companies, including Moongrove, which is working on no code interfaces for latent diffusion models, are striving to lower the barriers to implementing image generation models.
Ultimately, I imagine that just about any business process or consumer product involving sharing, creating, manipulating or showing images will be improved or enhanced by generative AI.
Prashant Fonseka is a partner at Tuesday Capital, an early-stage, sector-agnostic venture capital firm, and co-founder of Moongrove. At Tuesday Capital, Fonseka has backed Airtable, Airbnb, Uber, Cruise, and others.
Illustration: Dom Guzman
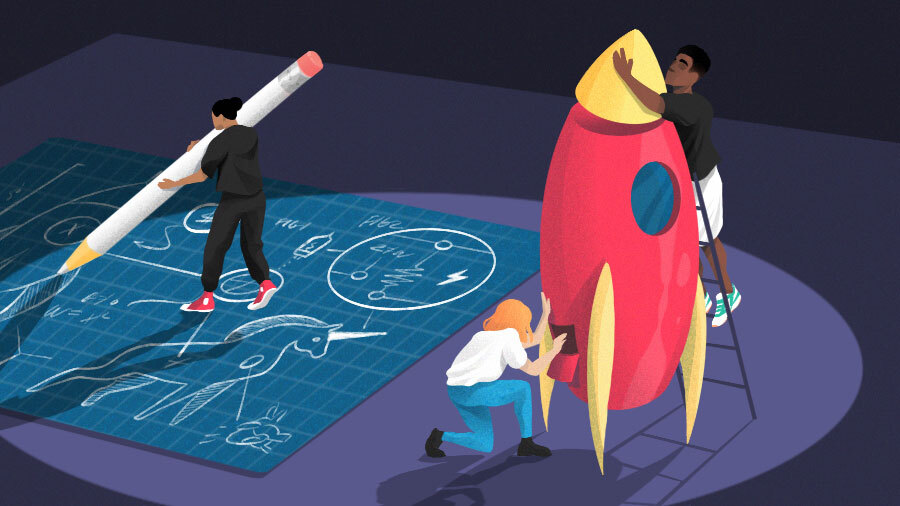
Stay up to date with recent funding rounds, acquisitions, and more with the Crunchbase Daily.
67.1K Followers